Introduction
AI for Emerging Markets is proving to be a game-changer in addressing the critical environmental threats faced by developing countries in Asia, Africa, and Latin America. These threats, which include climate change, pollution, and loss of biodiversity, are hindering progress towards sustainable development goals. However, the application of artificial intelligence offers genuine potential to mitigate these challenges through scalable and data-driven solutions specifically designed for the emerging markets.
One such solution is sensor-based waste and water monitoring mechanisms that leverage AI capabilities. These mechanisms enable real-time tracking and analysis of waste and water management, allowing for more efficient and sustainable practices. This technology has the potential to revolutionize waste management systems in developing countries, reducing pollution and promoting a cleaner environment.
Additionally, AI-powered satellite-based forest fire prediction models can play a crucial role in mitigating forest fires, a pressing issue in many developing regions. By analyzing satellite imagery and historical data, these models can accurately predict the occurrence and spread of forest fires, enabling timely intervention and prevention measures.
Furthermore, AI can support small shareholder farmers through climate-smart advisory systems. These systems utilize AI algorithms to analyze weather data, soil conditions, and crop cycles, providing tailored recommendations to farmers. By optimizing resource allocation and suggesting climate-resilient farming practices, AI-driven advisory systems can enhance agricultural productivity and reduce vulnerability to climate change impacts.
As the World Bank estimates, the impacts of global warming, such as extreme weather events and rising sea levels, pose a significant threat to the livelihoods of millions in developing countries struggling with poverty. However, with the leveraging of AI for emerging markets, these threats can be effectively addressed. By harnessing the power of AI, developing countries can implement scalable, data-driven solutions that contribute to sustainable development and environmental resilience.
However, barriers around skills gaps, poor data quality, and digital infrastructure limitations constrain the broader deployment of such AI applications for environmental conservation by Global South governments and enterprises. Although over 60% of citizens reside in developing countries, they account for less than 15% of global investments in AI, as industry reports point to lopsided access and participation divides. Surmounting adoption gaps necessitates cooperation frameworks to help diffuse green AI expertise between developing countries struggling with common ecological threats.
With astute policies and partnerships tailored for local limitations, AI could serve as a seminal technology reconciling twin vital goals of poverty alleviation and climate resilience for the 21st century Global South and help preserve fragile ecological habitats depleted by non-inclusive economic growth patterns. Leadership commitment and sustained investments into responsible frameworks remain vital for this.
Environmental Threats Facing Developing Countries
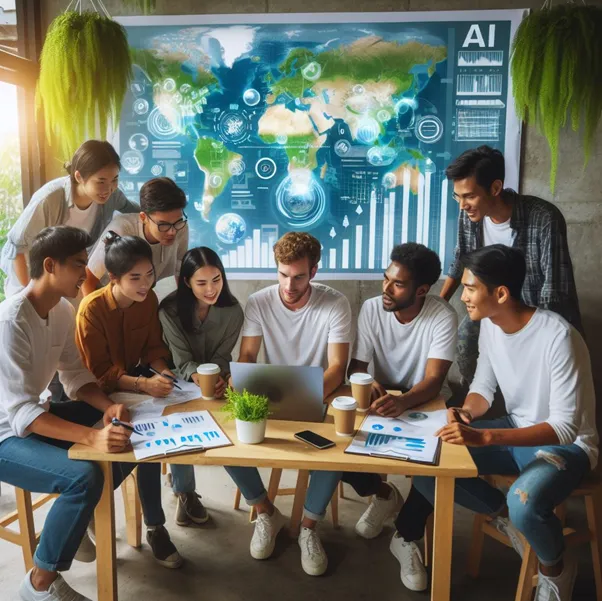
The developing world struggles with manifold environmental threats – air and water pollution, plastic waste, desertification, and deforestation – exacerbating public health crises, food insecurity, biodiversity declines, and climate vulnerability for billions struggling with poverty. Given low-lying geographies and economic reliance on climate-sensitive sectors like agriculture and forestry, global warming will impact developing countries disproportionately. However, low baseline environmental data availability exists, forecasting capabilities for evidence-based policy-making as countries grapple with more immediate priorities around income boosts and service delivery. Turning around these systemic ecological threats requires innovation around scalable, affordable solutions attuned to limitations of infrastructure and variability in socio-economic contexts across AI for emerging markets.
The Promise of AI in Tackling Sustainability Challenges
AI for emerging markets holds genuine promise as a seminal technology for transitioning developing economies faster towards climate resilience, optimized resource utilization, and delivering on sustainable development goals. Machine learning tools can analyze vast troves of earth observation data from satellite imagery and sensors to help with predictive analytics around ecological shifts and disaster forecasting – use cases pertinent for climate adaptation but requiring computing capabilities beyond the capacity of most government agencies in these nations. Using drones and cameras, computer vision can enable low-cost, consistent monitoring of environmental compliance, forest covers, and biodiversity protection. Automated waste sorting underpinned by deep learning categorizing different materials can drastically optimize recycling and treatment pathways in cities struggling with oceans of unmanaged waste accumulating daily.
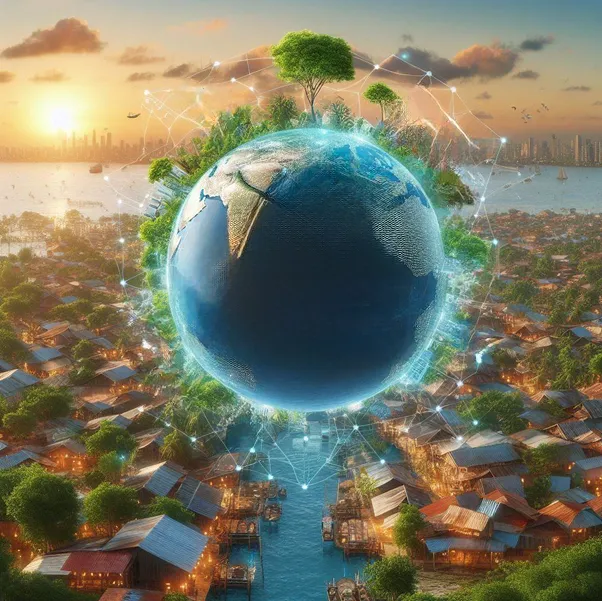
Internet of Things (IoT) devices with embedded machine learning are already helping modernize electricity grids and equipment maintenance protocols, lowering the carbon footprint of energy infrastructure. In Costa Rica, solar-powered AI listening devices deployed in rainforests track illegal logging in real-time, enabling faster conservation action. Agricultural AI applications provide personalized advisory to small shareholder farmers on sowing and pest management based on predictive analytics using historical and hyperlocal datasets boosting climate resilience and yields. Such innovations demonstrate genuine potential for AI to uplift sustainability in a socially inclusive, cost-effective manner if harnessed astutely.
Barriers Hindering Widespread Adoption
However, multiple barriers around data systems, digital skills, and unsupportive policy environments across developing countries constrain the accelerated adoption of AI solutions for environmental issues at national scales. Deficient environmental data collection practices need to capture sufficient indicators with a granular representation of diverse urban and rural contexts. Lack of digitization and interoperability between existing siloed datasets also hampers training robust machine learning models. Most projects remain small demo pilots powered by external grant funding rather than enterprise capital, limiting sustainability. There is a need for more specialist AI talent equipped with climate science domain expertise to architect tailored solutions. Coded biases can creep in unintentionally into supposedly neutral algorithms, affecting the fairness and accountability of environment-linked AI tools. Such limitations impede developing countries from harnessing the full potential of AI to enhance environmental sustainability across cities, farms, or industrial operations.
Strategies for Mainstreaming Environmental AI
Concerted policy efforts and public-private partnerships framing considered strategies for the developing world context can serve to more comprehensive mainstream AI solutions enhancing sustainability while balancing social welfare inclusion goals:
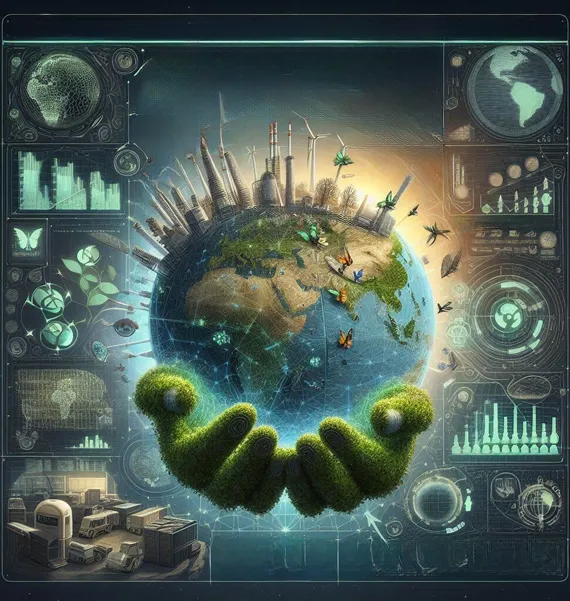
- Invest in climate-focused AI research networks: Set up dedicated university centers of excellence around use cases like climate modeling, agriculture, and disaster response funded by public and donor grants that can drive R&D while also training specialist talent in environmental AI attuned for constrained environments typical of the Global South.
- Support digital agricultural extension systems: Set up national digital repositories on weather, soil health, and crop parameters for location-tagged hyperlocal insights supporting farmer decisions on seasons and irrigation and create institutional frameworks for continual upgrades attuned to the needs of smallholder communities.
- Incentivize the private sector, especially around operations with climate impact, to invest in sensor analytics, predictive maintenance capabilities using tax breaks, procurement quotas, and making government data available through appropriate anonymization protocols for superior national forecasting.
- Responsible AI governance frameworks: Evolve sound data protection laws and transparency mechanisms, providing citizens visibility on how public agencies utilize algorithmic systems around areas like pollution violations and deforestation alerts while respecting privacy rights and human oversight over automation.
- Regional cooperation mechanisms can help share best practices between developing countries on fostering green AI skills, benchmarking data systems, and testing sandboxes adapted for local limitations to drive innovation.
Future Trajectory for Environmentally Responsible AI
The developing world is faced with twin, often conflicting priorities of uplifting millions out of poverty while preserving fragile ecological habitats historically depleted by non-inclusive economic growth. However, AI calibrated thoughtfully to balance access, transparency, and accountability goals offers renewed technological possibilities for achieving climate justice at the grassroots by empowering vulnerable communities with hyperlocal, contextual insights that can aid their resilience and lift environmental sustainability across cities, farms, and industries.

New machine learning applications are already attempting to rectify some of these historical limitations around nonrepresentative data and accountability concerns by incorporating inputs from indigenous and local communities in conservation efforts. However, sustained investments by the leadership of AI for emerging markets, robust data collection mechanisms, digital infrastructure adapted for the Global South, and incentives mainstreaming such solutions responsibly into both private and public sector operations remain vital for long-term, scalable impact marrying ecological stability, economic progress and community welfare. Overall, if harnessed astutely through cooperative strategies pooling insights across developing nations, artificial intelligence could serve as a seminal technology reconciling the twin vital goals of poverty alleviation and climate resilience for the 21st-century Global South.
Conclusion
Environmental sustainability challenges in the developing world require innovative solutions attuned to resource limitations and ground realities. With committed leadership steering considered benchmarking and policies tailored for poorer nations, AI holds genuine potential to transition these economies faster towards climate resilience, optimized conservation practices, and sustainable development – but this necessitates long-term investments in skills, data systems, and experimental cultures adapted for the Global South. Regional cooperation and open standards can also help diffuse green AI capabilities between developing countries struggling with common ecological threats. Artificial intelligence calibrated for inclusion and accessibility goals offers renewed possibilities for achieving climate justice and ecological stability across some of the world’s most vulnerable regions.